3 Technical Debt Metrics Every Engineer Should Know

Our team helps elite software engineering teams measure, prioritise and address technical debt. This article shares the best, tried-and-tested scientific methods to get quantitative data on technical debt.
Technical debt is an inevitable part of the software development process.
Modern software companies take on technical debt to enable them to ship faster. When we accumulate tech debt prudently, this makes sense. However, with technical debt comes technical risk. If it’s not managed, tech debt will impact every corner of your business, from team morale to customer satisfaction.
If you haven’t already read our Complete Guide to Technical Debt, we suggest you check it out.
Managing tech debt isn’t straightforward. In this article, we share the findings of the best researchers in the field so you can put them into practice.
This article will explore the three most important metrics for measuring technical debt: ownership, cohesion and churn. These metrics help engineers understand how to solve technical debt.
1. Measure ownership to prevent defects and unwanted tech debt
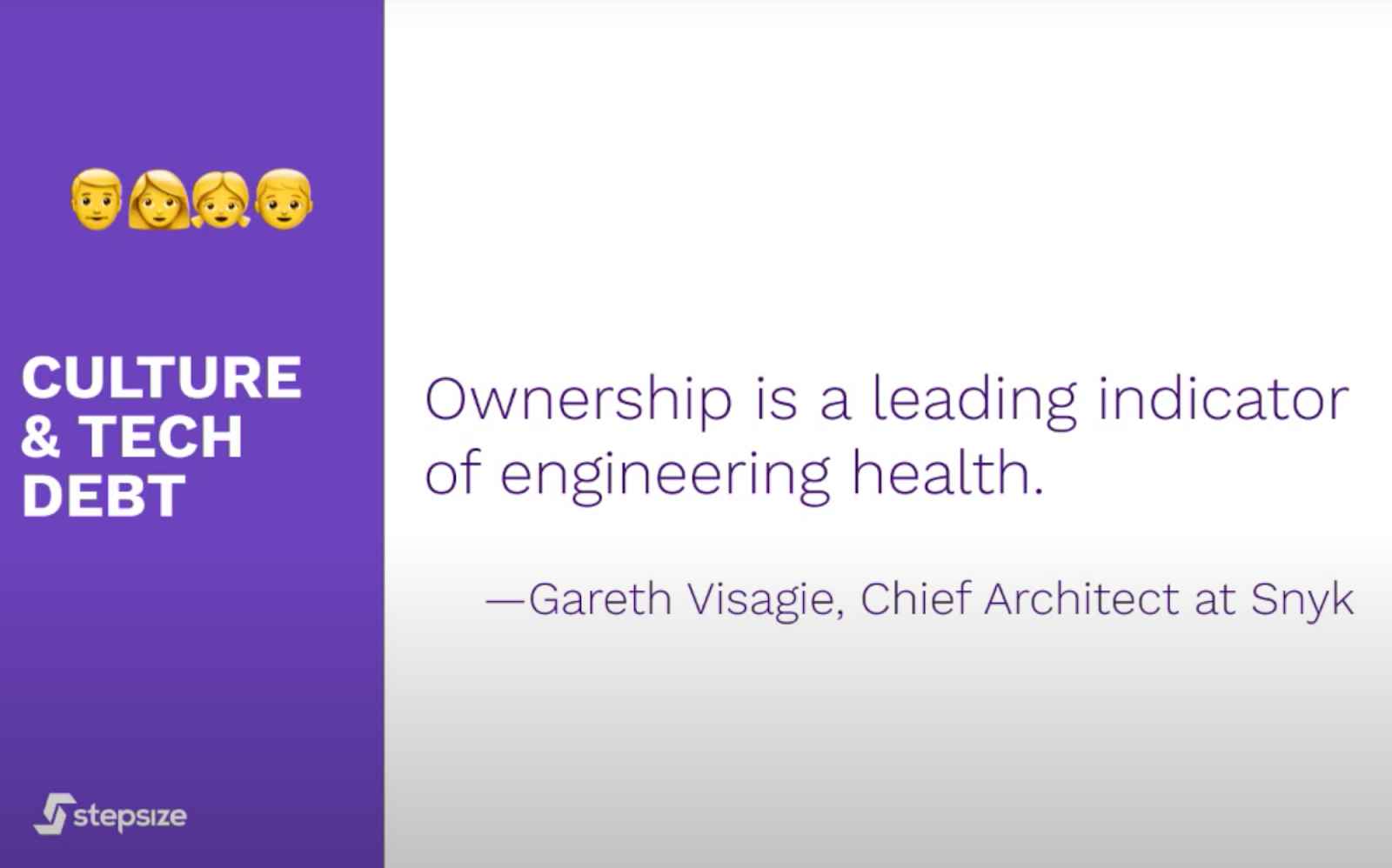
Measuring ownership is a meaningful way to prevent tech debt from accumulating. Areas with few contributors are likely to be in better shape, while those with many are more likely to accumulate debt.
Tracking ownership can help identify parts of the codebase that need extra attention. Ownership metrics allow us to predict that weakly owned parts of the codebase will cause more problems.
Top tip 💡Tracking tech debt using Jira can be painful. It feels clunky and disassociated with the code. Create and manage Jira issues directly from your editor by using Stepsize. Track and manage actionable issues that are linked to code, directly from the IDE.

How to calculate code ownership from Git data
To calculate code ownership, review the Git blame data of the current revision and examine the historical activity of each file. Blend these numbers while applying a time discounting factor to favour recent activity.
To determine “major” and “minor” contributors, set a threshold of 5% ownership. Apply a time discounting factor to favour recent activity when blending the two numbers. Aggregate code ownership scores over any subset of the codebase.
Academic research to back it up
You can read the full paper here: Don’t Touch My Code! Examining the Effects of Ownership on Software Quality — Microsoft Research, but I thought you’d appreciate a summary.
Microsoft explored two hypotheses on the Windows Vista & Windows 7 codebases:
- Hypothesis 1: Software components with many minor contributors will have more failures than software components with fewer.
- Hypothesis 2: Software components with a high level of ownership will have fewer failures than components with lower top ownership levels.
Both hypotheses were valid for the two codebases. While both minor contributor numbers and ownership levels had statistically significant impacts, the number of minor contributors had the largest impact.
How to use code ownership data to your advantage
Strong ownership should be the default for most codebases, with exceptions for situations with high uncertainty or experimental features. Strong ownership can help keep technical debt low for core codebases that power successful products.
What to look out for in code ownership data
1) Look out for minor contributors modifying code they shouldn't own
Identify minor code contributors who are not part of the ownership group and aim to reduce their contributions. Discuss the data with the team to determine the cause. Could it be indicative of poor architecture or lack of communication?
2) Make sure minor contributions are reviewed by major contributors
Maintain code quality by having major contributors review code from minor contributors. This helps catch mistakes before they are released to production. Code quality is a big topic – read more about it here.
3) Check whether functional teams own expected domains
Ensure the appropriate teams own codebase domains. Identify any domains with weak ownership and allocate them to the right people. Plan how to increase the strength of ownership for the future. For instance, the platform team should not be making changes to code related to payments, invoicing, and billing.
2. Measure cohesion to improve your architecture
Measuring code cohesion is an essential part of managing technical debt. Code is said to have high cohesion when most of its elements belong together.
High cohesion is associated with maintainability, reusability, and robustness. It also minimises the number of people needed to modify a section of the codebase, increasing productivity. Cohesion, along with its counterpart, coupling, should be evaluated when designing software to ensure the codebase is as efficient and effective as possible.
How to calculate cohesion from Git data
Measuring cohesion in polyglot codebases is difficult. To measure the cohesion of developer activity, look at Git data instead.
If a commit consists of changes to files within a given path, it is a cohesive commit. Cohesion is calculated as the ratio of cohesive commits to the total number of commits relevant to the path.
Academic research to back it up
You can read the full paper here: On the Relationship between Program Evolution and Fault-Proneness: An Empirical Study — Fehmi Jaafar et al.
This study examined the relationship between program evolution and the distribution of defects. They looked at Object Oriented codebases and found that classes with low cohesion of activity (i.e. those that co-evolved with others) had significantly higher numbers of defects than classes that evolved independently.
How to use cohesion to your advantage
High cohesion and loose coupling should be a goal for components of any well-architected system. We recommend tracking it for every component that makes it past prototyping stages.
The degree to which you try to optimise cohesion is up to you. Some instances of coupling might be tolerable, while others are suspect and likely the source of defects and team inefficiencies. But just being aware of coupling will empower you to make better planning decisions.
What to look out for in cohesion data
1) Increasing cohesion percentage is good
Increasing cohesion indicates that modifications to your component are increasingly isolated from changes to other components. That’s a good thing and something to shoot for.
2) Find components coupled together to inform refactoring
For a given component, understand which other components are coupled to it, and how strongly. Then find all the coupled activity to try to diagnose the root cause. For example, you might find out that a specific part of your notifications stack is coupled to a component of the message queue it shouldn’t know about. This will guide your refactoring efforts. Read our complete guide to refactoring here.
3. Measure churn to identify files that cause most bugs
As systems grow, they become harder to understand. When engineers have to modify many parts of a codebase to deliver a feature, it’s hard to avoid introducing side effects and bugs. Write code that brings closure to files, and rigorously review, test and QA churning areas.
To calculate code churn, measure the number of times a file has been modified, added, or deleted over a certain period. This will help you identify which files have the most activity and are most likely to cause bugs due to increased complexity. You can then prioritise refactoring these files and reducing their complexity to minimise the chances of introducing bugs.
How to calculate churn from Git data
Paths that haven't been modified in the past month are considered "stable". Paths that have been modified twice or more during the same period are "active". They become "recurrently active" when active for multiple months. Recurrently active files tend to be the most prone to bugs.
Top tip 💡Use Stepsize’s tech debt visualisation tool to spot problem areas. You can get a real-time visualisation using this tool. Learn how to get started with Stepsize here. It works with Visual Studio, VS Code, JetBrains.
This tool gives you an overview of your existing technical debt and makes it easier to decide which bugs to fix.

Academic research to back it up
You can read the whole paper here: Active files as a measure of software maintainability — Microsoft Research. Or check out the summary article.
Microsoft's study of six large software systems revealed that, despite only comprising 2-8% of total systems, active files accounted for 60-90% of all defects. This highlights the importance of QA, testing, and refactoring efforts.
How to use churn to your advantage
In a growing system, churn will help you identify the pieces of technical debt that are most pressing to pay off.
In a maturing or mature system, churn will help you identify the components to break down to minimise the surface area of change and optimise for stability.
In a legacy system, churn will help you identify the active components to plan how to phase them out.
What to look out for in churn data
1) Top churning files are your primary source of bugs
Examine the top churning files for your whole system as well as individual components. Use this to determine whether the activity is expected and desirable or whether it's a symptom of debt that should be paid back.
2) Ensure the review rate of changes to churning files is high
Ensure all changes to churning files are reviewed since they are strongly linked to defects.
3) Maintain the proportion of churning files within an appropriate range
If you're dealing with a growing system, maintain this metric to an appropriate level for your various components to ensure a sane surface area of change. If you're dealing with a mature or legacy system, look to minimise this metric at the system level to trend towards stability.
Closing thoughts
The effective management of technical debt is essential for the long-term success of any engineering team.
By understanding and measuring these three tech debt indicators – ownership, cohesion and churn – engineers can better assess the current state of their codebase. Then, they can take the necessary steps to reduce technical debt and ensure the long-term health of their codebase.
Ultimately, good tech debt management starts with tracking high-quality issues in the first place. If Jira is a source of pain for your engineers, use Stepsize to bring issue tracking where it belongs: in your codebase. Stepsize issues are inherently linked to code, breaking the spiral of low-quality issues and low fix rates.
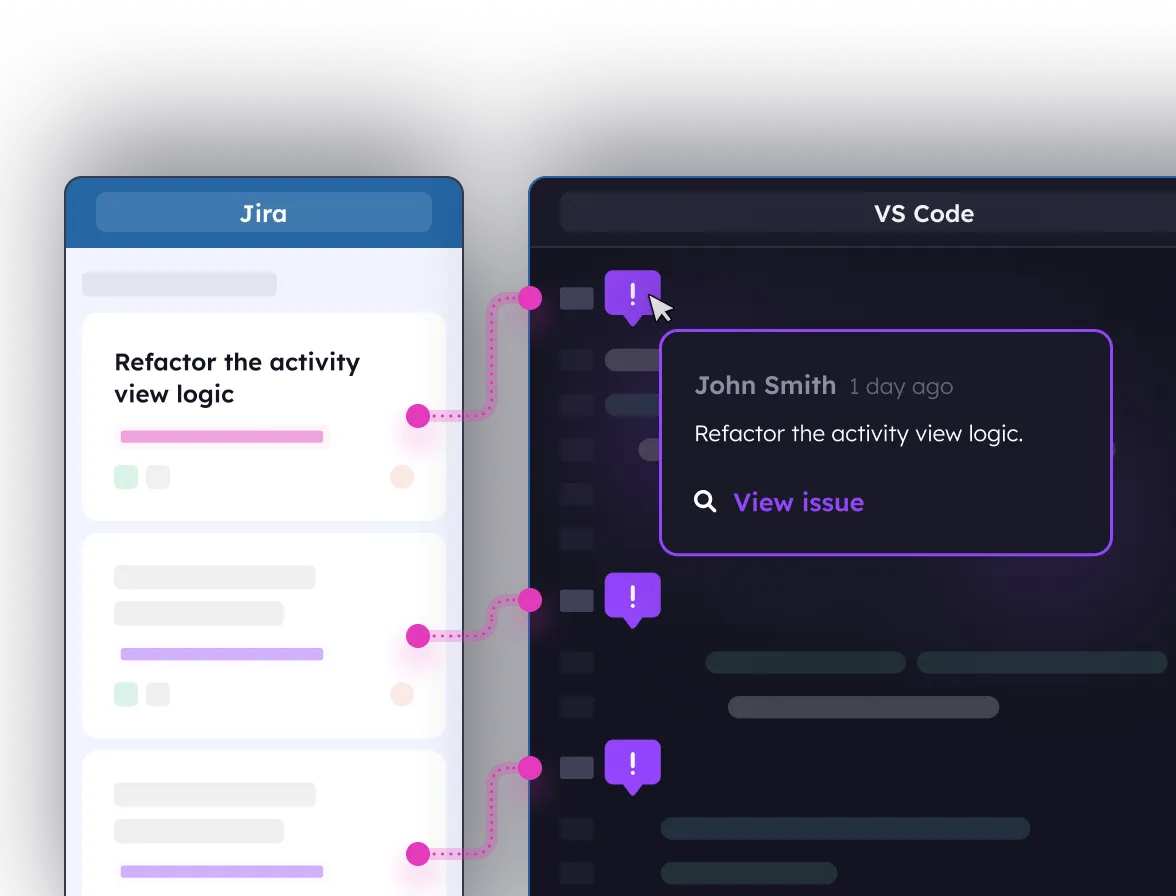
If you want to talk to an expert about technical risk, head here to book a call with our friendly team.